As service providers around the globe are doing their utmost to stay ahead of the curve when it comes to satisfying their customers’ hunger for bandwidth, the impact of this goes well beyond the data and control planes themselves.
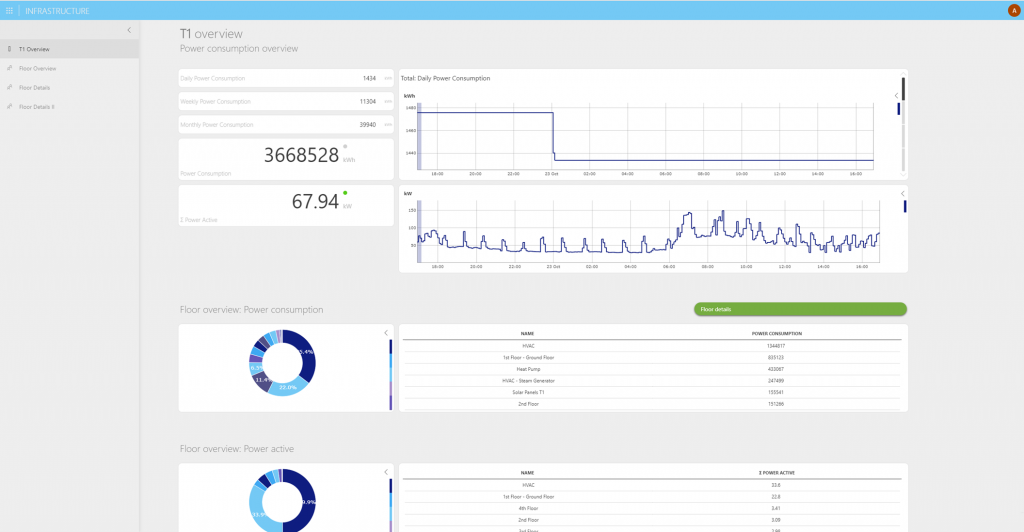
Delivering more than 1 Gbps to the end user, regardless of the access technology of choice, implies the distribution of high-capacity and power-hungry fronthaul/backhaul components and service nodes deep into the network. This also implies a multiplication in the number of sites that need to be powered.
It is quite common for an operator to only provide power to the bigger nodes, while relying on third parties to power all the smaller nodes. This leads to an increased concern related to the assurance of a correct and uninterrupted supply of power to the entire setup. And that is also what drives more and more operators to pay more attention to assure and optimize the energy supply of their network.
The goal of this DataMiner use case is to introduce important aspects related to the coverage, and to briefly introduce the options to approach this requirement as well as typical test protocols for some of the most common types of installations.
First of all, it is critical to cater for the requirements of the entire installation, including both:
- On-premises installations (e.g. datacenters or larger network nodes).
- Off-premises installations powered by a third party (e.g. outside plant cabinets or smaller network nodes).
A first approach is reactive. You tackle the outages and problems as they arise and strive to improve the installation as you go along.
- This is often the default approach with off-premises installations. Without access to the installations themselves, outage logs will be analyzed to conclude the runtime beyond the loss of the primary supply. That analysis serves to detect installations that perform below expectation and as such require e.g. batteries to be swapped or added.
- DataMiner Advanced Analytics has an important additional value by complementing straightforward ranking by runtime with correlations between performance and installation type, geolocation or utility provider. It also gives the opportunity to flag which systems fail to comply with the availability as agreed in the service level agreement (SLA).
Operators who deliver a premium or life-line service have little alternative than to opt for a proactive approach. It’s important to highlight that there are several differences both in the target as well as in the test protocols, depending on whether the installations are deployed “on- or off-site” and whether they’re “battery- or generator-based”.
The target for on-premises installations is to confirm whether the installations are ready to take over whenever a main outage would happen. For off-premises installations, the target is to capture information related to a planned outage and use that info to either provide advanced notice to the users as impacted or arrange for the temporary installation of a fallback system.
The protocol to assess the health status and capacity for on-premises battery-based systems is typically based on recurring partial and full discharge tests.
The partial discharge test probes for faulty batteries and returns the estimated runtime for the power supply using data related to the installation, battery types included, and the ambient as well as the load. This test detects the faulty battery and as such enables a quick replacement, but it also helps to detect whether a swap-out of an entire string of batteries is necessary, in case it shows a predicted runtime that is not acceptable.
A full discharge test is the only option to learn the actual runtime and as such crosscheck the findings from the partial discharge test. In contrast to partial discharge tests, which are common to be executed on a monthly basis, full discharge tests are likely to take place only once a year. The main reason for that is because a full discharge wears out the batteries and implies an operation without power back-up for the period of time it takes to recharge the batteries after the discharge.
Generator-based power systems require a given amount of fuel per hour and as such, the maximum runtime is set by the size of the fuel tank. The availability of this type of on-premises installations is typically captured over a periodic engine start. The capacity to meet the targeted runtime is assured by monitoring the level in the fuel tank.
It is clear that DataMiner is perfectly suited to cover for this energy supply assurance use case, but it is also important to mention that many customers report the same DataMiner setup is very effective in reducing their power bill.
That’s because the same DataMiner application as deployed for power assurance and optimization also tracks the load of the installations. DataMiner Advanced Analytics supports the automatic detection of e.g. level shifts or outliers in the load, enabling the earliest discovery of excessive power consumption associated with misalignments, faults or unauthorized power taps.
One of the most useful features of this solution is to know the actual impact on the network when a Power Supply is affected by a loss of AC energy.
With it, you can actually know what your network availability is and plan the batteries’ expansion/maintenance accordingly.
Great comment Arturo! I should have added this indeed. Thanks for adding this here. Dominique
Indeed Arturo, good point. Investing in battery backup without monitoring, especially in an HFC outdoor plant, is not exactly going to result in a solid ROI. Being able to efficiently coordinate interventions before batteries drain is a key capability. Battery capacity is also decreasing over the years, and this can be very different from one battery to another (i.e. depends on nbr of discharge cycles, depends on temperature fluctuations the batteries are subject to, etc.), so the software has to be intelligent enough also to asses that quality in order to make accurate forecasts.